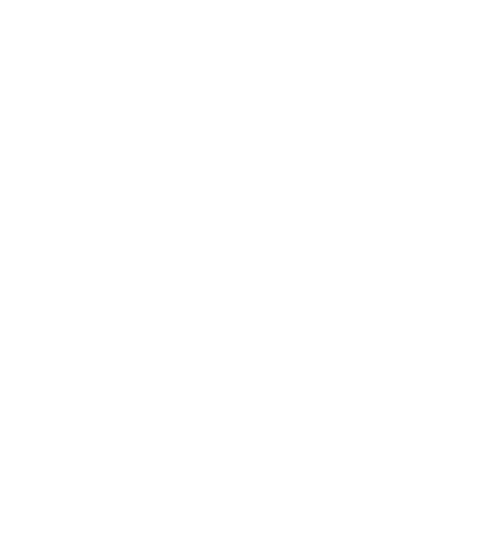
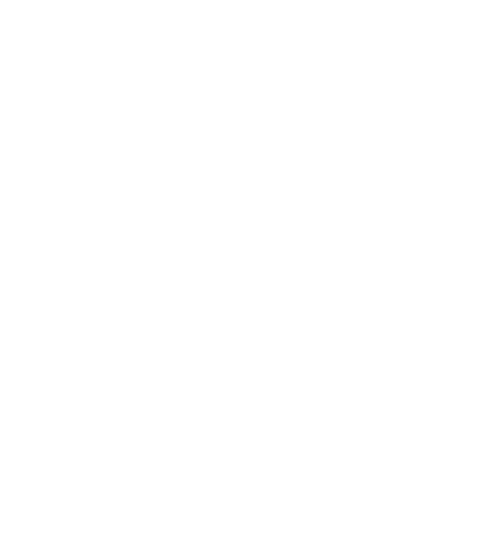
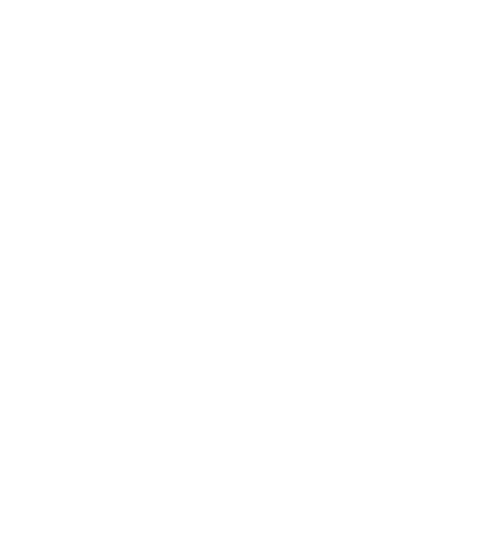
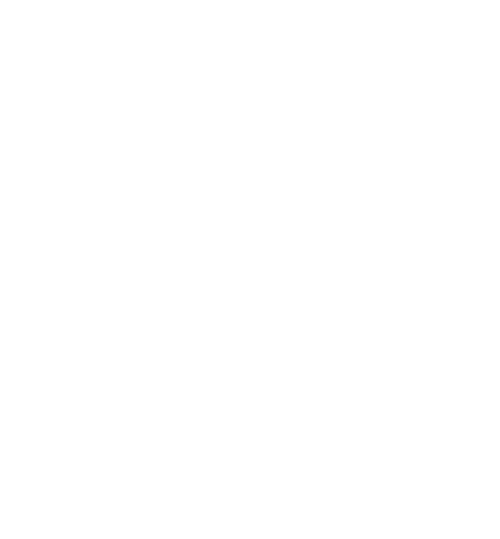
Symposium on "Forty Scientists Working Behind Closed Doors: Artificial Intelligence and Robots"
What challenges and opportunities will the development and application of robots face in the wave of artificial intelligence represented by large models? How will artificial intelligence technology and robots better integrate to meet market demand? Will intelligent robots that can understand, reason, and interact with the physical world be the future direction of robot development?
Around these issues, we held a seminar on "Science 40 Closed door Farming: Artificial Intelligence and Robotics" at the Greater Bay Area University (preparatory). The seminar invited Yao Xin, Vice President of Lingnan University and Tang Tianshen Chair Professor of Machine Learning, Wang Yu, Chair Professor of Greater Bay Area University (preparatory), Dean of the School of Advanced Engineering, and Chief Scientist of Dai Meng Robotics, and Sun Ruoyu, Associate Professor of the School of Data Science at The Chinese University of Hong Kong (Shenzhen) and Director of the Big Model Center at Shenzhen Big Data Research Institute, to jointly explore the opportunities, challenges, and future development trends of robot research in the era of artificial intelligence. The symposium was hosted by Tian Gang, president of Dawan District University (preparatory), chair professor of Peking University, and academician of the CAS Member. Xia Zhihong, chair professor of Dawan District University (preparatory), vice president of Dawan District Institute of Higher Studies, and editor in chief of Intellectuals. The following is a transcript, which is collated by intellectuals.
Tian Gang: Welcome everyone to the University of the Greater Bay Area, where 40 people from Science are working behind closed doors. The universities in the Greater Bay Area are still in the preparatory stage, but they have already made a good start. We have introduced a group of internationally renowned scholars and welcomed the third batch of students. The first section of the Songshan Lake campus project has been delivered. We have been approved for the National Natural Science Foundation of China in 2024, which is more than twice as much as last year. I hope that universities in the Greater Bay Area can receive continuous support from all sectors of society
Science Forty is a scientific exchange public welfare project initiated by some of our scientists, committed to becoming an advanced scientific exchange platform in China, focusing on scientific research, technology industry, and technology governance issues, actively promoting domestic and international academic exchanges and ideological collisions. The topic of today's closed door discussion among 40 scientists is artificial intelligence and robots. Artificial intelligence and advanced manufacturing are both areas of great concern for universities in the Greater Bay Area. We have established the Advanced Engineering College, led by Professor Wang Yu. We have also established an Intelligent Computing Research Center at the Greater Bay Area Advanced Research Institute, which is also related to artificial intelligence. I look forward to everyone colliding and sparking ideas.
Tian Gang, President of Dawan District University (preparatory), Chair Professor of Peking University, and Academician of the CAS Member
01 Popular challenges and scientific issues in the field of humanoid robots
Xia Zhihong: Firstly, please have three guests share their insights in their respective fields and introduce their research and applications in these areas.
Xia Zhihong: Firstly, please have three guests share their insights in their respective fields and introduce their research and applications in these areas. Professor Xia Zhihong, Vice Dean of the Greater Bay Area Higher Research Institute, and Editor in Chief of "Intellectuals" at the University of the Greater Bay Area (preparatory)
Yao Xin: In the vast field of artificial intelligence, I focus on a subfield called evolutionary computing. The core idea of evolutionary computing is very simple: many complex and sophisticated systems in nature, such as the human brain, are not artificially designed, but formed through evolutionary processes. Since such complex systems can be generated through evolution, there must be certain principles behind them that we can use to design better computer systems.
This idea can be traced back to a paper published by Turing in the journal Mind in 1950, which mainly discussed the Turing test, but Turing also spent three pages explaining how future computers would be programmed. I strongly recommend everyone to read this paper. It does not have complex mathematical formulas, but is full of inspiration. When you place yourself in the context of the 1950s, when most people knew nothing about computers, Turing's ability to propose such an idea is undoubtedly epoch-making.
In the field of evolutionary computing applications, my main research areas are related to optimization, including engineering optimization, digital optimization, and combinatorial optimization. Another important application area is evolutionary learning, which aligns with current machine learning goals but differs in implementation methods.
Yao Xin, Vice President of Lingnan University and Tang Tianshen Professor of Machine Learning
Wang Yu: Forty years ago, I went to Carnegie Mellon University in the United States to pursue a doctoral degree and began research in the field of robot operation. At that time, robots had not yet been widely used in industry, but the operation, movement, and mathematical applications of robots were considered very important and challenging scientific research work. Marc Raibert, the founder of Boston Dynamics, is a friend of my mentor and both of them came to Carnegie Mellon University. I started researching robot operation.
In the field of industrial robots, operation usually refers to using an end effector, such as a double claw or tool, to perform tasks such as grasping, welding, or riveting. The most universal and sacred manipulator is undoubtedly the five finger dexterous hand. Although we have made some breakthroughs in hardware after forty years of development, we have not yet achieved a qualitative leap in the control method of the five finger dexterous hand. We still lack effective mathematical and physical models to thoroughly solve this problem from an engineering perspective.
Professor Wang Yu, Dean of the School of Advanced Engineering, and Chief Scientist of Daimon Robotics at the University of the Greater Bay Area (preparatory)
Forty years later today, fields such as robot learning and big language modeling have become very popular. We have solved many problems in robot operation, movement, and vision, and believe that now it may be a core set of tools that we can see, which can truly combine computing methods, computing systems, and hardware to make the robot's operational ability reach a level close to that of human children. Although it cannot be said to have the operational ability of an adult, it can at least reach the ability of a child aged five, six, or ten to work in a workshop or at home.
Therefore, one of the hottest challenges in the current field of humanoid robots is to completely solve the problem of dexterous operation of robots, so that humanoid robots can bring value to humans like non humanoid robots. I have hope for this and believe that we have actually made quite good progress.
Sun Ruoyu: My research focuses on optimizing algorithms and neural networks. Initially, I focused on non convex optimization algorithms in machine learning. About seven or eight years ago, I noticed that neural networks are also non convex problems, so I joined Facebook AI Research to study neural networks. In recent years, I have mainly researched algorithms for large-scale models, including training, pre training algorithms, SFT (supervised fine-tuning) algorithms, and RLHF (reinforcement learning human feedback) algorithms. My goal is to develop more efficient and controllable algorithms for large-scale models.
Recently, I have been focusing on researching the issue of forgetting in lifelong learning and how to improve the efficiency of reinforcement learning. In the field of robotics and robot intelligence, big models are also a very hot topic, which may be an important direction for the future, similar to the "holy grail" of the field. At present, some of the main discussions in the field of big models, such as data scaling, synthetic data generation, avoiding pattern collapse, continuous improvement, and complex reasoning, are also important directions for the future development of the robotics field.
Sun Ruoyu, Associate Professor of the School of Data Science at The Chinese University of Hong Kong (Shenzhen) and Director of the Big Model Center at Shenzhen Big Data Research Institute
In the field of big models, our concerns also include how to perform complex reasoning, which is related to symbolic reasoning in AI for math, but also include the more difficult problem of how to reason in natural language, which has many solutions in the big model field. But in the field of robot intelligence, the situation is more complex because it also involves issues such as control, the combination of vision and language. I believe that in the field of robot intelligence, the exploration of these issues may have just begun. Although we see a glimmer of hope, I hope that these discussions can help us find a path to solving the problems.
Is the iPhone moment of 02 embodied intelligent robots coming soon?
Is the iPhone moment of 02 embodied intelligent robots coming soon? Xia Zhihong: The topic we are discussing today is related to embodied intelligent robots. At the 2024 World Robot Conference, which just ended in Beijing in late August, a total of 27 humanoid robots made their debut, setting a new record in history. Some people say that now is the "turning on time" for embodied intelligent robots; Some people also say that in a few years, there may be an iPhone moment with embodied intelligent robots, one for every person. What are the differences between embodied intelligent robots and traditional robots? How much longer does it take for an iPhone with a embodied intelligent robot?
Yao Xin: When we discuss embodied robots or humanoid robots, we need to consider the context more. My first reaction is that if we want to discuss this topic, we should first think about what tasks we hope these embodied robots can accomplish. I always feel that embodied robots are a means, not an end. Why do we need to create robots that resemble humans? What does' like a person 'really mean? Is it imitating human thinking or action? I think these are two completely different concepts, so I would like to bring up this issue for everyone to discuss. If we only discuss the phenomenon of embodied robots without paying attention to their purpose, the discussion may become scattered.
My interest in embodied robots has been around for a long time, especially considering controllers or large model systems. When they are embedded into a machine and embodied, what impact will this embodiment have on artificial intelligence systems? This problem has been troubling the field of evolutionary computing for thirty years. Will human form, animal form, and even robot form have an impact on brain structure or neural network structure? If so, what kind of impact would it have? In fact, early experiments and papers have shown that morphology and brain are inseparable.
However, currently there are relatively few scientists in this field who truly combine robot research with artificial intelligence research. If these two fields are developed separately, at least from a theoretical research perspective, it will bring many shortcomings. The structure of human neural networks is influenced by morphology. For example, we have symmetrical limbs, but if we have three heads and six arms, the neural network structure will be completely different.
From the perspective of embodied intelligence, I believe that more attention should be paid to two aspects: first, clarifying what we truly want robots to do; The second is to encourage universities to consider whether embodied intelligence has introduced new scientific and research questions, or whether it is just an application.
Wang Yu: The core value of humanoid robots or embodied intelligence lies in their ability to function as machines, with universality and versatility in partially controlled environments, capable of performing tasks and bringing true value to humanity. This value may be reflected in information processing, such as processing text and images, which is currently the work we are doing more in the field of artificial intelligence. And when we talk about machines with physical carriers, that is, reaching the level of embodied intelligence, it means that this machine can provide you with benefits and value, that is, it can perform actual work.
From the highest level perspective, an important issue is how to equip a machine with such capabilities. From the perspective of a machine, we are more concerned with its capabilities rather than its intelligence. In English, intelligence and ability are two different concepts, but in Chinese, the term artificial intelligence seems to confuse the two. At present, especially large language models and neural networks are considered the most promising tools, which enable machines to understand the environment, recognize the environment, understand instructions, and ultimately generate action rules, trajectories, and even operations and controls to complete tasks through perception and interaction with humans. From a practical and economic value perspective, this is the goal we must achieve.
In addition, when it comes to deeper issues, if a machine has certain forms of expression and capabilities, how will it affect the upper level neural system, computing system, network system, and neural network system? This is related to human development. I have recently discovered a very interesting phenomenon: if we study the development of human, especially operational ability, linguists will compare human operational ability and language ability on the same timeline. You will find that as human operational abilities improve, the richness of language, grammar, and vocabulary also increases. We don't know if this is accidental, but operational skills do stimulate brain development because as your needs increase, it stimulates the formation of neural networks.
Operational ability can also affect the overall deployment of the nervous system. For example, when you are laying eggs, cooking, or folding clothes at home, your brain hardly needs to think, and you can even think about abstract mathematical formulas while frying eggs. This means that much of our information processing is already done in our fingers, palms, arms, and even below the spinal nerves. Only when the environment becomes complex or urgent, do the brain's nerves begin to intervene. Therefore, with the improvement of operational ability, your nervous system needs to adapt to achieve the most efficient and energy-saving way of survival.
These are adaptations made by natural biological systems, and we are now beginning to raise such questions in artificial systems as well. Why do we need a large deep neural network and end-to-end computing power? For example, do I need to do this calculation from start to finish when I go to catch an egg? This is the least economical. Therefore, there are many very interesting questions worth exploring here.
Sun Ruoyu: At the level of embodied intelligence science problems, we can explore them from multiple perspectives. From the perspective of big models, one question is: should we only use language models as an interface to control robots, or develop "big robot models"? This involves a currently hotly debated topic in the field of big models: the existence or absence of world models. Some people believe that relying solely on language or textbook knowledge to learn cannot be connected to the real world. This involves the concept of grounding, which is how to connect abstract mathematical models or representations with representations in the real world. If this kind of connection can be achieved, then when developing robots and embodied intelligence, it is only necessary to ensure the correctness of the interface. This is a viewpoint.
Another viewpoint is that language learning alone is not enough, it is also necessary to learn visual world models. An example recently discussed is Sora, whether it has a world model. The mainstream view is that even if Sora has a world model, it is still very primitive. If that's the case, how can modeling of the physical world be achieved? As for language models, they rely on a large amount of data, such as 10 trillion data. But in terms of visual modeling, it may require 10 trillion video data. Where do these data come from? That's why many companies are generating video data and following the path of visual big models. This is a different possibility that I have seen technically.
At the level of embodied intelligence applications, an important question is what should embodied intelligence do? Embodied intelligence itself is just a form, not a goal. Goals can usually be divided into two types: To B and To C. To B may be related to intelligent manufacturing, while To C is home services. Most of the applications we see in the news are home services, such as folding clothes and Stanford's stir fry robot. From a technical perspective, an important challenge is generalization, that is, whether clothes can be folded in another scene after being folded in one scene. The challenge of robots providing services lies in whether they can provide services in 10000 different scenarios.
There may not be only one path for the development of intelligence, nor should there be only one
Xia Zhihong: Teacher Wang Yu once used a very vivid metaphor to describe the relationship between big models and artificial intelligence: big models are like brains, while the overall structure of robots, including human robots, is much more complex than brains. We may all have the experience that sometimes we understand many things in our hearts, but we cannot express them or do not know how to take action. Like a great novelist, he may be full of creative inspiration and impulse, but unable to translate these ideas into words. Robots are no exception, even if their "brain" is highly developed, they still require a "cerebellum" or a more detailed "midbrain" to coordinate and control their movements.
Another interesting point raised by Teacher Wang Yu is that our current model seems to first calculate on the machine, and then load these calculation results into the robot to perform the tasks we want it to complete, but humans do not work in this way. Taking tennis as an example, when you see a tennis ball flying, you need to quickly estimate its position and trajectory. For experienced tennis players, they are already prepared at the moment the opponent hits the ball, and it is no longer the brain that is at work, but the midbrain and cerebellum. Can Teacher Wang further explain our views on the brain, midbrain, and cerebellum?
Wang Yu: In the field of robotics, especially for us robot startups, a core issue is how to empower robots with intelligence to perform tasks and attract investment, ultimately achieving success in the market. This is a challenging technical problem, and different people have different perspectives. There are mainly two schools of thought: one advocates the use of large-scale language models and world models, integrating all possible physical phenomena, language phenomena, and visual information into a super large model, and processing problems in an end-to-end manner. This viewpoint is popular among young AI researchers and some top professors, especially at universities such as Tsinghua and Peking University.
On the other hand, people like us with white hair, because we have made robots before, we try to put robots in workshops and also try to put robots at home to wash dishes. We know how difficult it is, so we believe that intelligence should start from the basics and gradually expand to a wider range of applications. To enable robots to interact with humans, absorb information, perceive and process in a universal environment, they must possess intelligence. Therefore, tools such as large-scale language models have become crucial.
For example, if there is a service robot at home, when the owner says "I'm hungry", the robot needs to consider many things: is there a refrigerator at home? What food is in the fridge? What does the host like to eat? wait. But ultimately, when the robot needs to take out a plate, it involves its specific skills. I am currently promoting a concept called 'embodied skills', which refers to the ability of robots to perform specific tasks such as tying shoelaces, buttoning or screwing screws. These skills are usually acquired through learning and practice, rather than direct involvement of the brain.
Another important point is that when we enter a new environment, such as becoming a skilled worker, we usually receive training to learn specific skills. These skills are our potential abilities, not dependent on external design models. The key now is how to find an artificial intelligence learning method that allows robots to unleash their potential, learn and execute these skills. Of course, the hardware of robots must also have corresponding functions, such as precise finger control, rich tactile perception, and eye hand coordination ability.
Investors typically hope to see robots solve problems and demonstrate their abilities, but they often do not understand complex concepts such as the brain, midbrain, and cerebellum. They are more inclined to see large world models that are well done and able to solve problems. Although there is controversy, I believe that if these two paths continue to develop, there will eventually be a successful one, and things can come to a conclusion.
Xia Zhihong: Can Teacher Sun share on how to provide higher-level intelligent empowerment for already manufactured robots through machine learning and deep learning algorithms?
Sun Ruoyu: One direction is to introduce self-learning algorithms into robots. But recently I've come across an interesting question: even if robots or models can learn on their own, should we give them that opportunity? Some even suggest legislating to prohibit the self evolution of models. The reason behind this is the concern about uncontrollability. Nowadays, secure artificial intelligence is a very hot topic. Even for language models, how can we ensure that they do not say inappropriate words after continuous learning? If a robot can cut fruits, how can we ensure that it won't cut walls or harm humans? Before endowing robots with more capabilities through deep learning, we may need to address safety control issues.
Xia Zhihong: Teacher Yao, can we use evolutionary algorithms to further evolve pre trained robots and give them more abilities?
Yao Xin: Researchers in evolutionary algorithms believe that there may not be only one path for the development of intelligence, and there should not be only one. The current big model is basically based on the idea of collecting global data as much as possible. If the data collection is comprehensive enough, or even if it is not comprehensive enough, a huge model can be built through self generated data. This model is so large that it contains everything you can think of to solve problems. But researchers in evolutionary computing often believe that this concept implies an assumption that the world is static. However, in a dynamic and uncertain world, how can we ensure that the data we collect is comprehensive and accurate?
Researchers in evolutionary computing are more focused on how to deal with this uncertainty and dynamism. They believe that there is inherent uncertainty in defining problems, not just environmental uncertainty. To give an inappropriate example, in machine learning, many researchers spend a lot of effort designing loss functions, and once the loss function is determined, mathematicians will search for the optimal solution. But the problem is that once the loss function is determined, it cannot be changed during the machine learning process. However, in real life, the goals of many problems are difficult to formalize, and the loss function often changes during the actual learning process.
Therefore, researchers in evolutionary computing have been thinking about how to deal with these uncertainties and dynamics, which may be an interesting research topic. It is speculated that the emergence of brain regions in the process of biological evolution may have been to cope with temporal uncertainty and dynamism, such as the distinction between rapid and slow responses. Of course, these are currently inconclusive.
Why are robot hands inferior to feet and operations inferior to movement?
Xia Zhihong: We have been curious about robots since childhood. At that time, computers did not have intelligence, and we were very excited to see robot dogs or cats walking. Nowadays, the technology of robot walking is quite mature, but what I want to ask is, why can we make robots walk well now, but it is difficult for their hands to operate flexibly like human hands?
Yao Xin: If we go to the Science Museum in London, we will find a map that shows the mapping areas of various organs in the human brain, especially the motor organs such as hands and feet. In this picture, the hand occupies a huge area in the brain region. From the number of neurons and brain area, it is evident that controlling the hand requires more brain resources, which is an observed phenomenon. As for why hand control is so complex, biologists may need to provide more explanations.
The materials I have read indicate that hand control not only requires more degrees of freedom, but also that there is indeed a correlation between hand control ability and language development. It is currently unclear whether there is a causal relationship between the two, but correlation does exist. The reason behind this correlation may be a topic that model researchers need to further explore.
If this is true, then we can infer that the task of controlling the feet is to some extent simpler than controlling the hands, at least from the perspective of brain activity. This may explain why current robot technology for controlling the feet is relatively easier, while controlling the hands is more challenging.
When I was chatting with a robot researcher before, I joked that if you want to become famous overnight, make a robotic arm that can control chopsticks to pick up peanuts. I said, if you can do this, you will definitely make headlines because using a robotic arm to control chopsticks to pick up peanuts involves the integration of multiple senses such as vision, reasoning, and touch, which is a huge challenge. If you can integrate these controls into one system, it would be a remarkable achievement.
Wang Yu: The example of using chopsticks to pick up peanuts mentioned by Teacher Yao is a very exemplary robot operation task. Teacher Xia's question actually touches on the core issue of mechanical engineering, which is how to control a mechanical system with dynamic characteristics to achieve the expected performance. In the field of robotics, the main functions are divided into two categories: movement and operation. The development of mobile functions is relatively rapid, while the progress of operational functions is relatively slow.
Essentially, movement involves changes in the state of the robot system itself, such as position, velocity, and acceleration, without directly altering its environment. Although robots may encounter uncertainty and interference in their interaction with the environment, such as uneven ground or ice surfaces, their main task is to control their own stability. But the operation is completely different, it requires the robot to interact with the environment, especially using tools such as chopsticks, to change the state of objects in the environment, such as picking up peanuts or tightening screws. This involves complex interactions between robots, tools, and target objects.
For mobile robots, the objective function is usually clear: to maintain the stability of the robot's center of gravity, control its posture, and achieve the predetermined position and acceleration. All of these goals can be quantified and described to ensure that the robot does not fall or deviate from its path. Reinforcement learning, as a powerful tool, can handle these problems, while the underlying model predictive control provides precise action execution for robots. In terms of hardware, significant progress has also been made in the development of sensors and actuators, enabling robots to receive and process feedback information at speeds of kilohertz or even tens of thousands of hertz per second. The application of these technologies enables robots to perform complex actions such as flipping or jumping without losing balance.
However, in the field of robot operation, especially when it comes to tasks involving interaction with the environment, the problem becomes even more complex. The development of hardware is relatively lagging behind, and the most challenging aspect is how to define a suitable objective function to handle environmental uncertainty and interference. For example, for a robot, the task of fastening a button may seem simple, but during the learning process, it is difficult for the robot to learn effective feedback from continuous attempts and errors because throughout the entire process, unless the button is fully fastened, the feedback obtained by the robot will always fail, making the learning process very long and difficult. Therefore, how to design an objective function that can effectively guide robots to learn complex operational skills is a major challenge facing current robotics researchers.
In addition to reinforcement learning, there are also imitation learning, remote learning, and even some researchers have proposed "imaginative learning", which learns to complete tasks through imagination. If effective learning methods can be developed, it is believed that the operational ability of robots will develop synchronously with their mobility, which is exactly the issue that experts in the field of artificial intelligence should pay attention to.
Sun Ruoyu: Yann LeCun said that he prefers model predictive control over reinforcement learning, believing that reinforcement learning requires a lot of experimentation and is less efficient when learning new tasks, while model predictive control provides a more efficient solution.
One of the issues I've been thinking about recently is hand manipulation, and the complexity of grasping tasks is surprising. Despite numerous studies on grasping, this issue has not been fully resolved. The difficulty of grasping lies in the diversity of shapes, materials, and force control of the objects to be processed. Even the design of robotic hands has multiple forms, making the generalization ability of grasping tasks a key challenge. Humans have not seen all the objects they need to grab in their daily lives, but they can grab any new object they see. Where does this generalization ability come from? Generalization is one of the most difficult problems in machine learning, because to be honest, we are not entirely sure where the generalization ability of large models comes from.
Sanjeev Arora, a machine learning expert, proposed the concept of "skill mix" last year, emphasizing that large models have the ability to learn and combine skills. It is still unclear how to learn skill combinations in grasping tasks, but our understanding of the underlying mechanisms is still insufficient. Combining data and model predictive control methods may provide more efficient solutions for future research.
Can machine learning still generate true abstract thinking?
Xia Zhihong: In the field of machine learning, we put all the textual information we know into the computer and let the machine learn through probability theory. For example, when we input a sentence in ChatGPT, it calculates the most likely next sentence. However, the concept of probability seems to be opposite to human intelligence, especially the generation of innovation and inspiration. We usually believe that a person is creative because they have done something with a small probability, such as Einstein's theory of relativity, which was a small probability event in his time. Therefore, we often discuss intelligence and how to find things with small probabilities but significant impacts.
So far, the machine learning we have seen is all about probability events, which are things that most people can think of, but our capacity is not that large. I want to know, what do the three of you think about this? How can we enable machine learning to tap into this inspiration and find things that nobody could have imagined?
Yao Xin: In our previous discussion, several of our colleagues mentioned that current machine learning is somewhat similar to induction in mathematics. By observing a large amount of data, we can deduce some patterns, but these patterns are not true proofs, they may be correct or incorrect. Therefore, the purpose of machine learning is not to answer questions that require creative thinking, but to predict the probability relationship between future situations and previous data based on the massive amount of data it has seen.
At present, machine learning is unable to generate true abstract thinking. Although some studies claim that their models are capable of abstraction, this reminds me of the philosophical issues of the 'Chinese Room' in the 1980s and 1990s. From an external perspective, people may perceive machines as having or not having certain abilities based on their own perceptions. I believe that the ability to abstract and what kind of abstraction is more like a truth in real life may be closer to the small probability events you mentioned. Nowadays, large-scale models based on big data and computing power are not suitable for dealing with such problems. If you insist on letting them do such a thing, you may need to find other ways.
Xia Zhihong: Is it possible to improve existing machine learning methods so that they are not limited to finding high probability events, or can they discover less obvious and more innovative solutions while focusing on correlations?
Yao Xin: To achieve this goal, we need to propose different research questions, which should be different from the current issues of concern in the field of machine learning and take different paths. I cannot say exactly what it should be, but what I can be certain of is that the direction is different.
Xia Zhihong: My confusion lies in people's understanding of intelligence. Can induction be considered intelligent?
Yao Xin: I think this issue involves both scientific aspects and public perception. Usually, when someone presents the right idea that I didn't think of, I naturally assume that person is very intelligent, which is an instinctive reaction. But upon careful consideration, this situation may not be entirely a manifestation of intelligence, sometimes it's just because we haven't seen it. In my opinion, more valuable intelligence should be able to extract abstract concepts from observations, and these concepts should be consistent with the truth of the real world.
Xia Zhihong: So originality is usually something that machine learning cannot learn, right?
Wang Yu: This question was actually debated by pioneers in the field of artificial intelligence. The most famous discussion took place at the Dartmouth Conference in 1968, where many founders of artificial intelligence attended. At that meeting, different terms such as "expert system," "knowledge system," and "symbolization" were proposed. In the end, it was Marvin Minsky from MIT who proposed the term 'Artificial Intelligence'. Although he received criticism from some colleagues for this choice, believing that the term was not accurate enough, it was eventually widely accepted and circulated.
I believe that the essence of artificial intelligence lies in its inductive ability, which is the ability to extract patterns from existing data and predict what may happen next based on them. However, artificial intelligence lacks creativity because it cannot detach itself from raw data.
I particularly dislike the images generated by Sora. I believe that when we step into nature, climb mountains, and appreciate the scenery, what we see is the truly creative beauty of nature, and nature is constantly changing. The images generated by Sora, although visually appealing, do not have true innovative significance. I think this is a very fundamental issue because current neural networks do have significant limitations and cannot reach true levels of innovation.
Sun Ruoyu: This question is very mathematical, and I also respond in a mathematical way. Machine learning typically learns high probability events, but when we pose a correct problem, under the conditions of that problem, low probability events may become high probability events.
For example, taking mathematicians in history as an example, they sometimes put forward groundbreaking ideas, such as Riemann's famous speech on geometry. If we give artificial intelligence a task and simply ask it to give a speech, then what it gives is likely to be a mediocre speech. However, if we ask a specific and detailed question, such as asking it to give a speech that no one has thought of in the past 100 years, can create a new chapter in the history of mathematics, and contains some completely new ideas, when the qualifier is long enough, this small probability event - that is, the generation of innovative ideas - may become a high probability event.
Xia Zhihong: This is the application of "prompt" in artificial intelligence. In chemical pharmaceuticals, we have countless chemical reactions with the goal of achieving a specific outcome. If you already know what results you want, artificial intelligence can help you find chemical reaction pathways that could have been small probabilities. As long as your prompts are precise and appropriate enough, artificial intelligence can play a role in this process and achieve some valuable results.
Wang Yu: Suppose I give a specific prompt and say, "Now we have quantum mechanics, which is a very mature theory, right? At the same time, we also have Einstein's theory of relativity, which you have already understood. Einstein spent his whole life trying to unify these two theories. So, please tell me, what kind of result would be obtained if these two theories were combined
Xia Zhihong: This may require true intelligence, not artificial intelligence. Even if we optimize the prompts, the computer itself still needs to know how to explore. If the prompt changes and points to a field that the computer has not previously encountered, then it has no path. The application of artificial intelligence in the pharmaceutical field actually knows a path. It knows this small probability event, it's just verifying which of all the small probability events can lead to the ultimate success. This raises an interesting question: we humans are group animals and enjoy discussing together, but can you imagine future robots coming together, colliding and discussing with each other, and generating new ideas?
Sun Ruoyu: At the ICML conference in July, there was an article that was rated as the best paper, which explored what results could be achieved through bold debate. Although it remains to be seen whether such debates can generate new ideas, at least this topic has already begun to be discussed by people.
Yao Xin: Regarding prompts, if I provide sufficiently intelligent prompts, even large models can exhibit intelligence. That's not because the big model has intelligence, but because my prompts have intelligence.
As for the interaction between multiple intelligent agents, I believe it is entirely possible to generate a solution that does not belong to the original domain of these agents. Anyway, no matter how big a large model is, it always has a specific domain or domain of definition. If intelligent agents come from different domains and engage in debates, there will definitely be an interval that is not within their common intersection. Within this range, there is a possibility of generating new ideas, and I believe this probability exists.
Sometimes there may be innovative ideas that a single robot cannot think of. This raises a slightly philosophical question, similar to the "Chinese Room" paradox, which requires a "god like" presence to judge whether these ideas are useful, as intelligence is often related to practicality or whether they conform to physical laws. At present, intelligent agents are unable to make such judgments on their own, usually requiring a third party. Sometimes we may use the quoted word "God" to describe it, but in reality, it refers to humans.
Is humor and emotion a human privilege?
Xia Zhihong: In the field of artificial intelligence, we often hope that it can mimic human intelligence. Recently, I read an interesting article with a photo attached. In the photo, President Obama secretly steps on an official's weight scale from behind, causing the official's weight to show an increase, while Obama and his entourage are laughing happily.
When such photos are input into an artificial intelligence system, whether the machine can sense the humor and recognize the elements of humor is a question worth exploring. Previously, I always thought that this was an advanced perception ability unique to humans, not something that robots could experience. But recently, I heard that ChatGPT 4 was able to analyze this photo and point out all the humor, including officials not wanting to gain weight, Obama's behavior of secretly increasing weight, and officials' knowing smiles. I was very surprised by this, as I did not anticipate that existing large models could achieve such an effect.
This raises a question of whether artificial intelligence will gradually approach human levels in emotional understanding and humor. Can they become companion robots in the future?
Yao Xin: If we follow the idea we just discussed and integrate all the data in the world, including various information on the internet and books, into artificial intelligence systems, it is very likely that such machines will become closer to humans in understanding and expressing emotions, because these data contain rich emotional information.
However, the brain is responsible for processing many things. I read an article on evolutionary biology in Scientific American magazine, which mentioned that human intelligence is a "desperate solution". Why do you say that? Because in the biological world, humans do not have an advantage in many aspects, such as not being able to run as fast as leopards, having inferior vision to eagles, and having inferior smell to dogs. In the competition of real life, humans were originally unable to survive, and wisdom was forced out. In other words, intelligence evolved for survival, and first you have to survive, everything else is secondary.
The article proposes some hypotheses later, suggesting that certain structures and instinctive responses of the brain are hard coded rather than inferred. For example, when one sees an abyss, they instinctively avoid jumping down, which is not something learned. Why? Because those who tried to learn are no longer there, only those who instinctively avoided danger survived.
So, when we consider human intelligence, we may need to take into account the history of evolution, and not mistake some simple instinctive reactions, those already fixed behaviors, for those that can only be obtained through data learning. Genetic information not only includes a large amount of data before an individual is born, which is already built into our system, but also involves big data in the process of evolution.
Therefore, I believe that learning should consist of two stages: one is learning within an individual generation, which is very similar to the current process of machine learning; The other is long-term learning across multiple generations, which is more like an evolutionary process. If both dimensions of learning over a long time span and learning through big data in a short period of time can be taken into account, we may receive different answers to the question you just raised.
Wang Yu: The ability of GPT is indeed remarkable, and the success rate of ChatGPT 4.0 has significantly improved, partly due to extensive human intervention and correction. Compared to ChatGPT 3.0, version 4.0 has added more manual labor to optimize answers.
From a biological perspective, intelligence is indeed an interesting topic. Taking language as an example, MIT professor Noam Chomsky was the first to propose that language ability may be inherited. His initial viewpoint was that language ability is innate, not just acquired through learning. Now, this theory has been widely recognized by linguists.
Some extreme examples are children who are deprived of their right to speak at a very young age, such as being kidnapped and locked in a cabinet without anyone to talk to. When they were rescued around the age of 16, they could no longer learn to speak, no matter how they were taught. These unfortunate examples confirm that language ability is partially innate, but it cannot be fully developed without postnatal learning. For example, if a child starts learning a language later, their development speed may be slower, but if they start learning early, they can master the language.
The development of skills is also about being able to operate various tools and equipment. Is this ability innate or hereditary? This is difficult to prove because there have been no research cases of children being deprived of their operational abilities. Of course, such an experiment is too cruel, and we would not conduct such an experiment.
We also see that parents nowadays hope their children can learn various skills as early as possible. Naturally, the effects of children's learning from a young age are evident. Children can do this and be capable of that at the age of 3, all of which are the result of postnatal learning. However, without a natural genetic foundation, these complex systems and skills cannot be acquired through learning.
Sun Ruoyu: Many abilities are indeed inherited, and there are two stages: pre trained and trained. After thousands of years of evolution, we undergo a long pre training process before birth, where large amounts of data are integrated and learned, and then implanted into our genes. There is a book called 'Whiteboard' that specifically discusses this issue and explores Chomsky's viewpoint that people are not born on a blank sheet of paper.
Regarding the issue of emotions, I have a vague feeling that some simple tasks, such as buttoning or those on the production line, may actually be automated later. On the contrary, emotional companionship may arrive earlier in the field of robotics. The emotional intelligence of big models is actually good, not only in terms of humor, but also in terms of situational awareness, analysis of employee psychology, and emotional companionship.
For example, I often consult ChatGPT about issues related to colleague disputes, asking ChatGPT to analyze the psychology behind people's conversations; Then send a screenshot of the answer to a colleague, who often says that their analysis is very accurate. Emotional intelligence, or the ability to provide emotional companionship, may be achieved earlier, but the prerequisite is to ensure safety. Perhaps you remember last year's example where someone had an emotional breakdown after chatting with ChatGPT. If security is properly controlled, emotional companionship is possible.
Especially in this era, many people often feel lonely and need companionship. A young person from Generation Z told me that he would rather spend money online to chat with ChatGPT than date in real life. He believes that ChatGPT is better than real people, always willing to respond to requests and understand everything. So, emotional companionship may come earlier than we expected.
Xia Zhihong: I used to firmly believe that humans have souls, and artificial intelligence can never possess this. But as I delved deeper into psychological issues, I gradually realized that many psychological problems are actually caused by chemical imbalances in the brain. After appropriate medication treatment, these psychological problems can often be alleviated. This makes me wonder if human emotions, including humor, are also the result of chemical reactions rather than the embodiment of the soul. These ideas have left me particularly disappointed, but I am still willing to believe in the existence of the soul.
What kind of question is being asked in 07 and why is it being asked?
Xia Zhihong: We are discussing these issues at universities in the Greater Bay Area because the universities hope to make achievements in talent cultivation, especially in the fields of artificial intelligence and robotics in the future. I would like to ask everyone to discuss which direction and areas we should develop towards?
Yao Xin: I hope my graduate students can learn three things: firstly, to acquire new knowledge, and secondly, to learn how to ask questions.
I often joke that it has been 50 years since the reform and opening up, why are we still solving the problems raised by others? This may mean that we still lack the ability to ask questions. Now everyone has realized this, and we have also started hosting conferences to raise our own questions. But sometimes, the questions we raise seem to go unanswered. No matter how difficult the problem is, people are solving it with great enthusiasm 300 years ago. So we have to ask, why did later generations help solve the problems 300 years ago, but no one paid attention to the questions we raised?
So, in addition to learning knowledge and asking questions, it is important to learn what kind of questions to ask and why to ask them. The last point may be the most important.
The same applies to the field of artificial intelligence. Most people are making big models, but I am doing evolutionary calculations. If you make a big model and spend ten minutes a day thinking about evolutionary computation, maybe evolutionary computation can also be beneficial. By asking a few more questions, you will find that all roads lead to Rome, and artificial intelligence is not the only path to take.
Wang Yu: In the rapid development of artificial intelligence and robotics technology, my experience is that we need to promote interdisciplinary education in engineering. The development of modern technology indicates that the importance of hardware is increasingly prominent and rapidly advancing. At the same time, software, artificial intelligence tools, and computing technology are all complementary. Hard, soft, information, and control have been closely integrated together. If we are limited to the narrow field of traditional engineering education, such as the turning, milling, planing, and grinding that I learned when studying mechanical manufacturing in 1982, it will appear very limited.
Therefore, interdisciplinary and cross disciplinary education is particularly important, especially for students in engineering disciplines. There also needs to be a practical engineering application foothold, that is, whether we can ultimately transform what we have learned into products with commercial or technological value. This means that innovation and entrepreneurial spirit should also be a part of our education.
Sun Ruoyu: University education should combine new ideas with foundational fields. In the field of artificial intelligence, many people's shortcomings or limitations lie in their lack of solid foundational knowledge. For example, leaders in the field of artificial intelligence are familiar with model predictive control, but if you ask current AI researchers, many of them may not have delved deeply into basic courses such as optimization algorithms and linear algebra. Current education urgently needs to integrate cutting-edge technologies with foundational knowledge.
Xia Zhihong: While conducting research, universities also hope to translate research results into practical applications, which is particularly important for universities in the Greater Bay Area. We have established a research institute with the hope of using this platform to transform basic research results into products with practical value, and thus contribute to the socio-economic development. I believe that Professor Yao Xin also has a similar goal at Lingnan University, as the Vice President of Research, to focus on the issue of national achievement transformation. May I ask, what are the current directions worth paying attention to in the development of the robotics and artificial intelligence industries?
Yao Xin: In terms of knowledge transfer and application, there have always been efforts from two directions or two groups. A group is concerned about the major challenges facing society, and they will organize teams to tackle these challenges, which naturally leads to interdisciplinary cooperation. This group has a very clear problem orientation; Another group is also common in universities, who are committed to improving their research achievements and finding places to implement them. I think both approaches are necessary, as focusing only on one type of problem may sometimes harm divergent thinking.
But the second method has its limitations and may lead researchers into the trap of "finding a nail with a hammer", and in academia, whenever a new trend emerges, the second method is often preferred. For example, the role of artificial intelligence in solving social problems is often vague. Although we can see that chatting and pictures are quite fun, the actual effect of improving employment rates or solving other social problems is not very clear. Pushing the research direction towards practical applications is much more difficult than pure research work.
Taking Lingnan University as an example, as the Vice President of Research, I may be more inclined towards the first approach, as Lingnan University has traditionally been a liberal arts university that emphasizes humanistic care and education that serves society. The talents we cultivate should be able to identify social issues and contribute to solving these major problems. Therefore, whether it is conducting research, innovation, or knowledge transmission, we all start from this perspective.
As for specific social issues, such as social inequality, poverty, or security concerns brought about by artificial intelligence, once we identify these challenges, research and industry university research cooperation will revolve around these issues. This is at least a commonly used method at Lingnan University.
Xia Zhihong: Mr. Wang Yu's team has achieved a lot in terms of achievement transformation and innovation. What advantages do we have in the entire Greater Bay Area? What other aspects need improvement?
Wang Yu: The Greater Bay Area has a strong industrial foundation, which provides excellent conditions for the development of hard technology. Especially for technology holders who hope to transform basic technology into products, the supply chain here is very powerful, allowing for rapid prototyping and iteration. Compared with Europe and America, Shenzhen, Dongguan and other places undoubtedly have the best iteration ability and conditions in the field of hard technology in the world.
If teachers have innovative ideas and technologies and are willing to try commercializing them, they can take advantage of the environment of the Greater Bay Area to give it a try. However, entrepreneurship is a challenging process, and while we can encourage students and teachers to try, we also need to establish a certain support system. For example, schools can provide tutoring, training, and science and technology innovation training classes, and even establish science and technology innovation colleges to help people understand the process of knowledge transfer and technological innovation, as well as possible troubles and even pitfalls.
These preparations are very important, otherwise blindly guiding teachers and students to start businesses may lead them into difficulties. My own entrepreneurial experience started with blind experimentation. At that time, as long as you dared to try in China, you had a chance to succeed, but at the same time, you might encounter many pitfalls and have to find ways to get others to take you out. Now, with the development and standardization of society, relevant systems and systems are gradually being established, which will reduce the chances of failure. But we still need to make everyone aware of this and provide necessary support.
At present, universities generally recognize the importance of this link, but not everyone can fully understand it. The worst-case scenario is that every teacher has a hammer and goes out every day saying, 'I need to find a nail, find a nail, find a nail.'. This method is not the most effective and is also detrimental to the growth of students.
Xia Zhihong: I have noticed that Professor Sun Ruoyu has also ventured into many application fields, especially in the field of artificial intelligence and algorithms in the field of communication, while conducting algorithm research. Does Teacher Sun have any further innovative considerations in this regard?
Sun Ruoyu: In the past year or two, I have been exploring the application of artificial intelligence in various industries and have also discussed financing with some investors. The key question is which specific industry problems can be solved by current artificial intelligence. Hong Kong Zhongshen and Shenzhen Big Data Research Institute have been exploring what practical problems big models can solve. We have released medical big models, Arabic big models, and are also developing big models in the legal field.
But that's still the question, after releasing these models, we need to ask which pain point in the industry they solve. During the financing process, investors often ask this question. When we discuss embodied intelligence, we not only need to talk about big models with investors, but also discuss which parts of production, manufacturing, or services can provide solutions.
At present, I believe there are opportunities in the field of embodied intelligence, but it may take another two to three years to overcome technical challenges, find requirements, and ensure that the hammer (technical solution) matches the nail (actual demand).
Xia Zhihong: What else do the three guests need to add?
Yao Xin: When discussing research in various fields such as artificial intelligence and embodied robots, we often carry the personal imprint of scholars. This imprint is mainly reflected in a tendency to start from one's own professional field and think about which scientific problems can be solved, rather than starting from the problems themselves. Therefore, what I would like to call for is that when starting research, it is important to first clarify which scientific problem you want to solve and engage in in-depth discussions around it, which will be more targeted and meaningful. Otherwise, the discussion may become too scattered.
Wang Yu: At the August Robot Conference, a total of 27 humanoid robots were exhibited. Our Damon robot did not participate in the exhibition, and if it does, it may become the 28th, but considering the cost involved, we believe the timing is not yet ripe. What I want to emphasize is that technology is developing rapidly, with waves emerging one after another. For us, it is more important to cultivate critical thinking, to be able to deeply understand and judge, rather than relying solely on self promotion through self media. Our company's self media content, of course, always says that it's better if we have more.
For young people, it is very important to be able to grasp themselves and have the ability to critically view and think about problems, so that they can make correct judgments and decide what they should do next. Otherwise, sometimes one may be misled and lead towards less than ideal directions.
Sun Ruoyu: When discussing robots, more attention should be paid to specific application scenarios. A month ago, I attended a seminar on embodied intelligence where robot manufacturers discussed the challenges faced by their robotic arms and humanoid robots. I am very interested in this field, and for me, the biggest question is whether artificial intelligence can solve these problems and how long it will take - whether it will take 3, 5, or 30 years.
08 Audience Questions
Audience 1: Hello teachers, I am from Macau Polytechnic University. I have summarized two questions about artificial intelligence and robots. First, let me briefly introduce my thinking background.
Firstly, I am more inclined to adopt a robot development method that separates perception, control, and decision-making, rather than using a new type of large model controller technology. I have encountered a very tricky problem, which is whether there is a universal solution or method to control different types of robots, whether they are humanoid robots or other forms of robots. For example, humanoid robots have two end effectors, how can we coordinate and control them to complete the same task. From a control perspective, is there a universal solution that can be obtained by inputting basic information such as the robot's dynamic model, structure, and joint degrees of freedom.
Secondly, when robots operate objects and interact with the world, they will inevitably collide or pick up objects, which will cause changes in the environmental state they are in. Is there a brain like approach that can effectively understand and process models of relationships and interactions between objects. As far as I know, my understanding of large models in three-dimensional space is still limited, so my question is whether there are feasible solutions for these two problems.
Wang Yu: When discussing the movement and operation of robots, we can observe that if it is only movement rather than operation and does not involve the collaborative work of both hands, then through model predictive control and reinforcement learning, the control problem of robots has been basically solved. Now, robots can autonomously learn forward kinematics and inverse kinematics without the need for us to write traditional kinematic formulas. These technologies enable robots to autonomously control themselves, completing movements such as flipping and following.
However, when it comes to manual operation or simpler tasks such as grabbing objects with five fingers, twisting screws, or picking up peanut beans with chopsticks, the problem becomes more complex. At present, the development of collaborative operating systems remains an open problem, which is one of the most important challenges in the research of robotic and dexterous hands. We need to find a way for robots to master these skills through learning.
Who is the target of learning? Where does the data come from? Obviously, we cannot learn from elephants because their operating methods are too different from those of humans. Therefore, our only choice is to learn from humanity. We don't investigate why human hands have such a structure, because as engineers, our goal is to create robots that can have a wide range of operational functions like humans. By learning human abilities, we can transfer these skills to machines, which is called skill learning.
In fact, humans have strong adaptability. Even if they unfortunately lose their fingers, people can usually regain their ability to operate. Some people can even perform delicate actions such as grabbing spoons, chopsticks, and even sewing clothes with their feet. These examples demonstrate that human potential is enormous, and our goal is to tap into this potential and apply it to robotics technology.
As for why humans have five fingers or why they choose this type of hand structure, we may never be able to give a definite answer. Sometimes, we can attribute it to religious or philosophical views that believe it was created by God, or from an evolutionary perspective, it is the result of evolution for survival and more efficient use of resources. But regardless of the reasons, we can use these features to design and improve our robotics technology.
Audience 2: Hello teachers, I am a doctoral student from a university in the Greater Bay Area. I have two questions to ask, Professor Wang and Professor Yao respectively.
Teacher Wang, yesterday I visited an exhibition hall in Shenzhen where there is a coffee robot made of a robotic arm. It performs very well when it's not busy, but once it gets busy, coffee spills everywhere, making the environment very dirty. This reminds me that if humans make mistakes during the operation process, such as encountering simple situations, they may wipe them with their hands. And robots only focus on their goals and are not very concerned about the problems that occur during the process, as long as they complete the task. If a robot makes a mistake, who should be responsible for correcting it? In more serious cases, such as our current autonomous vehicle, if there is a problem, should the developer bear the responsibility?
Teacher Yao, you mentioned that there are many paths to evolution, and there is not only one path for us to achieve the ultimate goal of artificial intelligence. Just like when we play games, there may be many different endings, both good and bad. My question is whether we need to adopt some methods or means to avoid risks, in order to ensure that we can ultimately achieve a good outcome rather than a bad one. thank you.
Wang Yu: The first question you raised actually involves the behavioral performance of artificial intelligence. From my perspective, this is not directly related to the robot itself. If a robot causes chaos during operation, such as coffee spilling, it is not the robot's own problem, but rather the lack of guidance on how to clean it up. If a child accidentally spills something when they are young, if they do not receive timely education and correction, if their mother does not spank them, and if they do not reinforce their learning, they may not learn how to handle it.
Sun Ruoyu: This problem is actually something that Artificial General Intelligence (AGI) needs to solve. Traditional AI often focuses only on a single task, such as asking a robot to pour coffee, and it only knows how to pour coffee. And humans are universal, so when we perform tasks, we don't just do one thing, but handle multiple tasks according to the situation, which requires multitask learning.
In practical situations, it is difficult to define all possible tasks in advance. For example, when hosting a meeting today, if the microphone suddenly breaks, it involves another task. Therefore, the concept of big models is to enable AI to learn thousands of different tasks so that it knows how to respond when encountering new scenarios. So, this is indeed an AI problem that involves how to make AI more adaptable and capable of learning.
Yao Xin: Regarding the topic of playing games, I would like to make two responses.
Firstly, from an evolutionary perspective, there is indeed significant uncertainty and randomness in our ability to evolve to the current state. Every step in the process of evolution is full of contingency.
Secondly, when we talk about bad outcomes and good outcomes, there is an underlying assumption that there exists an external 'God' to judge what is bad and what is good. However, in natural evolution, there is no such criterion for evaluation. The only criterion for evolution is survival. If a species can survive, then it is successful; If it cannot survive, then it will be eliminated.
There is a fundamental difference between evolutionary computation and true biological evolution. All biologists will tell you that computer simulated evolutionary algorithms are unreliable because they misunderstand the true meaning of evolution. In evolutionary algorithms, we usually set a fixed fitness function, while in nature, the fitness function is survival. So, only individuals who survive can be considered excellent.
Therefore, if you understand these two points, you will understand that although all roads lead to Rome, if you ultimately go to Paris instead of Rome, unless you have an external observer to tell you the direction, you cannot avoid such a result. In evolutionary algorithms, we do have an objective function to guide direction, but in nature, we cannot fully express this preference with a formula.
Audience 3: I am a physics major and currently working in a company. In enterprises, our expectation for robots is that they can help us solve various problems. But in practical applications, we have encountered challenges in terms of generalization ability and self-learning ability. I have two questions to ask: First, how long do you expect generalization ability and self-learning ability to be resolved? Secondly, if these two issues are resolved, does it mean that robots will also possess innovation and originality capabilities?
Yao Xin: My first reaction was that I couldn't answer, one of the reasons being that your question wasn't expressed very clearly. When you mention solving generalization problems, I need to know specifically what you mean because generalization problems may have different mathematical expressions in different application scenarios. If you write out the formula, I should have a solution. But whether the method is good or bad, it may not be guaranteed.
I often find that in cooperation with the industry, many problems cannot be solved, not because the problem itself is too complex, but because the problem is not expressed clearly. Therefore, as university professors, we often need to spend a lot of effort helping companies clarify the definition of problems when collaborating with them. Once the problem is properly defined, researchers at the graduate level or above are usually able to find solutions, although these solutions may not be perfect.
So, I suggest you could provide a more detailed description of your application scenario and what level of generalization ability you are referring to.
Audience 3: Tools developed for specific scenarios, such as a simulation tool I previously developed that can automatically generate simulation scenes and run them in conjunction with the program. This is a completely customized design. But if the problem changes or the program needs to be adjusted, it often requires reconfiguring the tool. Fortunately, the configuration of this tool is relatively simple and can be flexibly adjusted. Can generalization also be achieved through configuration in the field of robotics or artificial intelligence? This is the problem we are currently facing. Many artificial intelligence systems perform well in solving problems in specific scenarios, but their performance in other scenarios is not satisfactory.
Yao Xin: I can provide an example that involves a specific field in evolutionary computing, known as experience based optimization. In this field, researchers design algorithms to solve the Traveling Salesman Problem (TSP). Now, the challenge they face is how to apply the algorithm for solving TSP problems with slight adjustments to address facility layout issues. This sounds very interesting because the algorithm originally used for path planning is now being used to handle allocation problems.
Currently, some researchers are exploring this field, but the results are inconsistent. Their goal is that algorithms that solve a problem can not only handle similar problems, but also differ in terms of coordination. Now, researchers are taking it a step further, hoping that the same algorithm can learn and solve different types of problems. This means that algorithms should be flexible and alive, rather than rigid.
This is somewhat similar to the idea you mentioned, that is, after a system is applied in Dongguan, it may only require some adjustments to the parameters in Shenzhen to be used, without the need to start from scratch.
Audience 4: In my exploration over the past few years, I have identified three common difficulties in the process of transforming scientific and technological achievements. Firstly, from new discoveries in academic research to the formation of theories, then to the application of knowledge and the presentation of preliminary results, ultimately achieving industrialization, this process is very long and full of unforeseeable factors, which may affect the success rate of our transformation. Secondly, when we are in the financing stage, investors usually pay close attention to the maturity of the product, which often determines whether they are willing to invest. Thirdly, my experience in the UK has taught me that scholars there tend to focus more on academic research and may not have enough time and resources to engage in in-depth industry academia research collaborations. Based on these difficulties, what are the real pain points in the process of transforming scientific and technological achievements in China?
Wang Yu: The transformation of achievements is indeed a complex process that requires multiple mature conditions to proceed smoothly. Firstly, the maturity of technology is crucial, and we typically evaluate it based on the Technology Readiness Level (TRL). A TRL below 3 indicates that the technology is still in the basic research stage, while a TRL of 3 to 9 indicates that the technology has certain potential for application. Only when it reaches levels 9 to 12 can the technology mature enough for industrial promotion. Therefore, we must have a clear understanding of the TRL level of our technology. If the technology has not yet reached a level that can be converted, attempting too early may waste resources and time.
Secondly, we need to clarify what problem the technology is solving. For example, even if you can manufacture a robotic arm, what problems can it solve? This involves identifying market pain points, which is the most difficult part in commercialization and investment. The business model and market path of the product will become more complex, requiring in-depth research whether it is aimed at consumers (2C), enterprises (2B), or governments (2G).
Starting a business and ultimately succeeding is not just a problem that academia can solve, nor is it a standard answer that any textbook can provide. It requires you to have the corresponding abilities, knowledge, judgment, and the ability to find the right team, seize opportunities in the right environment, and avoid getting stuck in difficulties during the process in order to succeed.
However, even if successful, maintaining the advantage over others is still a challenge. In the current fiercely competitive market environment, once a product is proven profitable, there will soon be numerous competitors flooding in, and the market will quickly become a red ocean. Due to strong domestic production and technological capabilities, as well as abundant talent reserves, once a product is proven to have a market, competitors will quickly organize the supply chain through dismantling and imitation, followed by price wars, market competition, and policy competition. Therefore, this is an extremely complex process.
Nevertheless, our market is huge, Chinese people are diligent and keen on innovation and entrepreneurship, which makes the transformation of achievements still worth trying. Most teachers focus on their own research work, and I also hope to excel in my research. However, at this age, I may not be able to prove more theorems, but I can use my experience and influence to lead my students and postdoctoral team and help them push their research results to the market.
Note: Science 40 is a scientific exchange and public welfare project jointly initiated by the Intellectual Frontier Technology Promotion Center of Haidian District, Beijing (referred to as the "Intellectual Research Society", produced by "Intellectuals" and "Mr. Sai") and the Zhejiang Kehui Zhiyuan Public Welfare Foundation. The Scientific Committee of 40 currently includes 34 top scholars from different disciplines. The seminar, "Science 40 People Cultivate Behind Closed Doors: Artificial Intelligence and Robotics", was co sponsored by Dawan District University (preparatory) and also supported by the ByteDance Public Welfare Foundation in Beijing.